· 9 min read
The surprising resilience of criminal organizations: new insights from complexity science
Small criminal groups are like tiny marbles easily nudged between valleys. As groups grow, they become like bowling balls, settling deep and resistant to disruption.
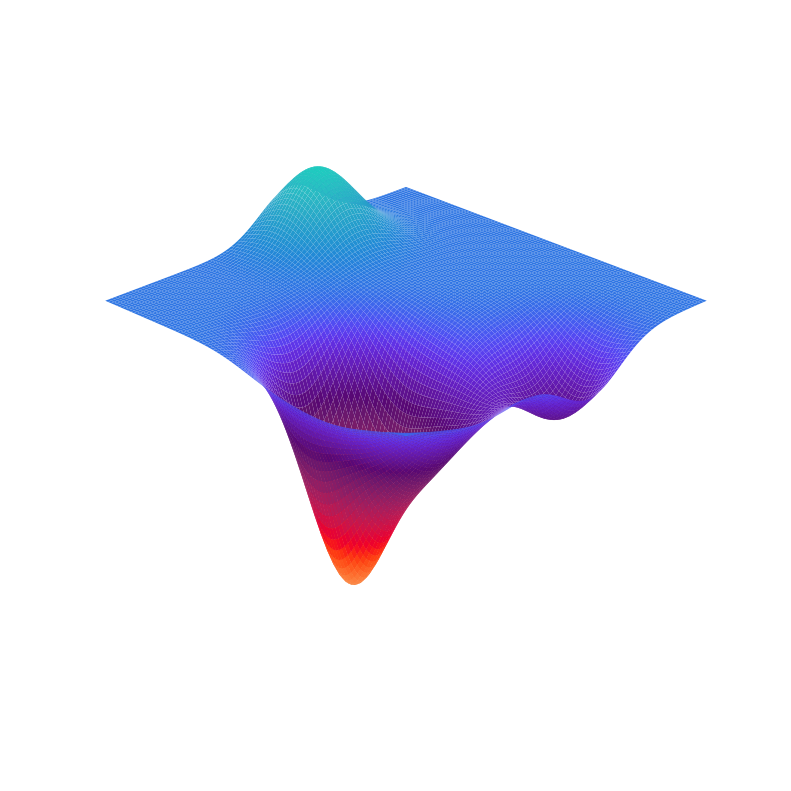
Law enforcement agencies and policymakers continue to grapple with a fundamental enigma in their battle against organized crime: What enables criminal networks to endure and flourish despite concentrated efforts to eradicate them? This question resonates across the criminal spectrum—from neighborhood street gangs to complex international syndicates. These illicit organizations exhibit remarkable adaptability, consistently evading permanent disruption in ways that have confounded authorities for generations. Could there be an alternative framework for understanding and addressing these resilient criminal ecosystems that might yield more effective intervention strategies?
Moving beyond static network analysis
Studying crime presents unique challenges. Criminal organizations operate in a constant state of flux, engaging in an intricate cat-and-mouse game with law enforcement and rival groups. Their adaptive nature, responding to both internal dynamics and external pressures, makes them particularly elusive subjects of study. Traditionally, researchers have approached criminal organizations from a network perspective, focusing on the “who-knows-who” aspect. Static network analyses, while informative, fail to account for the ever-changing landscape of relationships, roles, and strategies that define these adaptive systems.
Criminal organizations are far more complex than mere connection maps. They represent a sophisticated interplay between individual decision-making processes, group dynamics, and responses to environmental pressures. To truly understand and effectively combat these organizations, we need to move beyond static network models and embrace more sophisticated computational approaches. These computational models allow for testing ‘what-if’ scenarios to see how criminal organizations evolve over time.
The power of computational models
Researchers from the Computational Science Lab at the University of Amsterdam have developed a mathematical and computational model that simulates the formation and behaviour of criminal organizations. Their findings, published in Nature Scientific Reports, reveal how these networks become remarkably stable and resistant to external pressures once established – a phenomenon known as hysteresis.
“We were surprised to see how difficult it becomes to disrupt a criminal organization once it reaches a certain size,” says lead author Casper van Elteren. Imagine a landscape with hills and valleys, where criminal organizations are represented by balls of different sizes. Small criminal groups are like small marbles that can be easily nudged from one valley to another or even out of the landscape altogether. However, as these groups grow, they become more like larger, heavier bowling balls.
Once a criminal organization reaches a critical mass, it’s analogous to a bowling ball settling into a deep, wide valley. It becomes incredibly difficult to dislodge. Minor disturbances, which might have derailed a smaller group, now barely cause it to wobble. The organization has essentially created its own stable ecosystem within this valley.
The study modelled criminal organizations as a regular business. These businesses operate through networks of individuals with different roles, such as producers, distributors, and managers. Each “agent” in the model decides whether to engage in criminal activity based on potential costs (like the risk of arrest) and benefits (such as financial gain).
Strong hysteresis in the forming of criminal organisations
One of the most striking findings is that increasing punishments or costs for criminal activity isn’t always effective. Once a criminal network is well-established, it can withstand significant increases in risk or reductions in profit before collapsing. This helps explain why simply increasing prison sentences or police presence often fails to curb organized crime.”There’s a tipping point,” explains van Elteren. “Below a certain level of criminal activity, small changes can have big effects. But once you cross that threshold, the system becomes much more stable and resistant to change.”
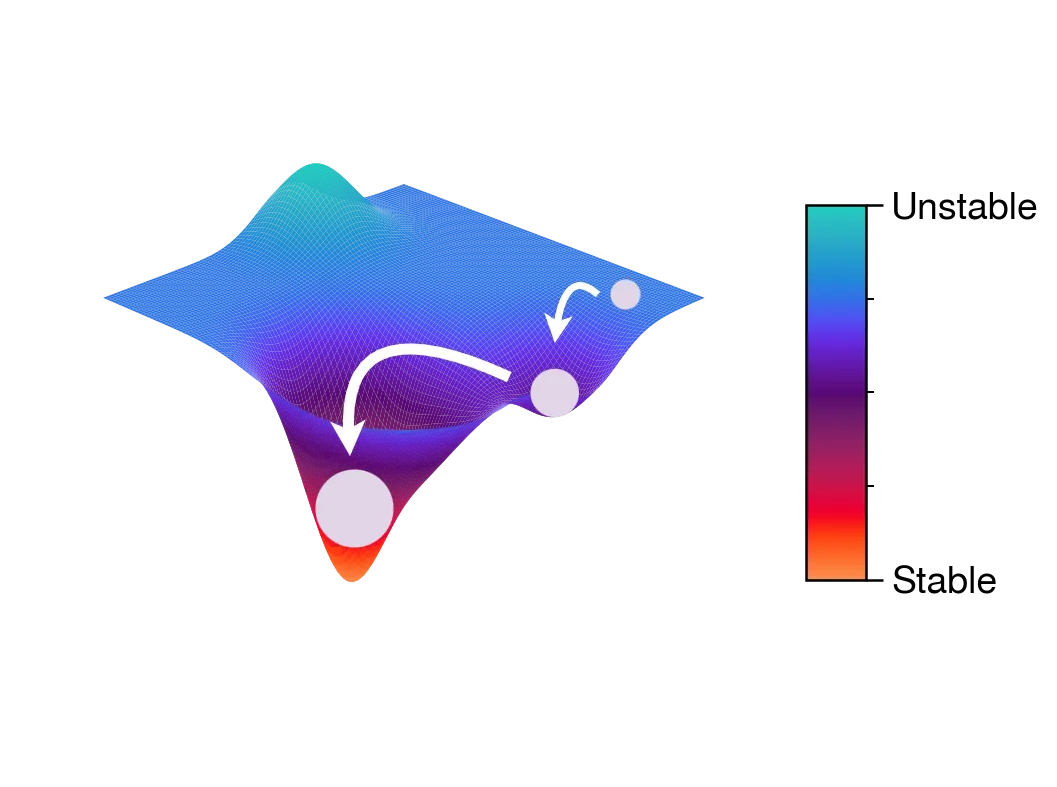
Figure 1. The resilience and adaptivity of criminal organizations can be likened to a marble moving over a surface. Some places are unstable where small nudges will move the marble down – akin to being on top of a hill. Stable areas are indicated by deeper troughs, making them harder to dislodge. When the criminal organization grows –akin to having a larger marble – it will be harder and harder to dislodge criminal organizations within deeper valleys. Perceived cost of the criminal act will dictate the shape of the landscape, creating pockets of criminality or making them disappear.
This concept, called hysteresis, sheds light on the challenges of breaking down established criminal networks. In physics, hysteresis describes systems whose current state depends on its history. The researchers found that criminal networks exhibit a similar property. “A smaller marble can be easily moved around navigating the highs and lows of a rolling landscape” explains van Elteren. “Once the organization has grown to a large bowling ball that settles into deep valleys, making them much harder to dislodge it especially when it is in a deep valley.Criminal organizations work the same way.” This means that the effectiveness of interventions depends heavily on context. In low-crime areas, a slight increase in policing may be enough to prevent criminal organizations from forming. However, in high-crime areas with well-established networks, the same approach has little effect. This hysteresis effect not only explains their resilience—how they recover from losses—but also their robustness, or ability to adapt to external changes.
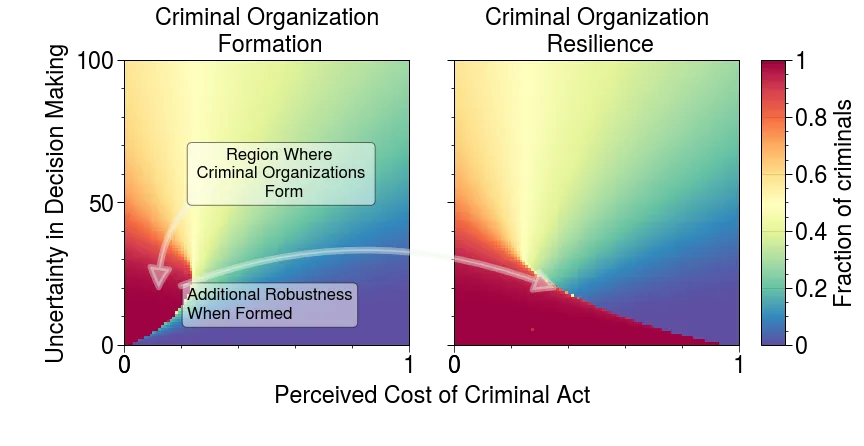
Figure 2. Criminal organizations gain strong resilience after being formed. Simulations were performed starting from two distinct scenarios. The first scenario (left) describes the situation in which the simulation starts without any criminals. When the perceived cost of a criminal act is low, criminal organizations spontaneously emerge as the perceived cost approaches zero. This effect becomes more pronounced as actors have incomplete information about their surroundings. With mild to moderate amounts of decision error criminal emergence. In the second scenario (right), the simulation starts with all agents as criminals. A wide tail can be observed where for increasing perceived cost, criminal organizations remain active. This increased resilience shows that criminal organizations gain resilience after being formed; when a criminal organization spontaneously forms in society (left) it “moves” into a regime in which further interventions does not yield the same result (right).
Credit. Scientific Reports
The devil you know
Interestingly, the model shows that the structure of social networks plays a crucial role in this stability. These networks thrive when there’s a high density of connections between individuals with specialized skills, enabling them to replace lost members and adapt quickly to change.
It’s not just about having a lot of criminals,” says co-author Vítor V. Vasconcelos. “It’s about having the right mix of skills and a network that allows for efficient collaboration while maintaining secrecy.”
Another critical factor is information flow. When criminals are well-informed about each other’s roles and the state of the organization, it becomes easier for the network to coordinate and maintain stability. This suggests that disrupting communication channels could be a valuable strategy for law enforcement. However, the study’s findings aren’t all bad news for those fighting organized crime. The model reveals critical points where criminal networks are most vulnerable:
Prevention is key: It’s much easier to prevent a criminal organization from forming in the first place than to dismantle it once established.
Targeting network structure: Focusing on disrupting the connections between different specialized roles within the organization can be more effective than simply arresting individual members.
Reducing benefits: While increasing punishments has limited effect on established networks, reducing the profitability of criminal activities can be more impactful.
Exploiting uncertainty: Increasing the “decision error” – the uncertainty criminals face about costs and benefits – can make networks less stable.
A complex system’s approach to fighting crime
“Our work shows that we need to think about fighting organized crime as a complex systems problem,” says co-author Mike Lees. “Simple, linear approaches are unlikely to work against these adaptive, resilient networks.”
While the researchers acknowledge that their model simplifies real-world criminal organizations—which are often more intricate and hierarchical—it provides valuable insights for shaping more effective law enforcement policies.
“This study demonstrates the power of applying complex systems thinking to societal problems,” van Elteren concludes. “By understanding the underlying dynamics of how criminal networks form and persist, we can develop smarter, more targeted approaches to combating organized crime.”
The team plans to refine their model with real-world data and explore how different intervention strategies might play out in various scenarios. They hope their work will contribute to a more nuanced understanding of criminal behavior and lead to more effective crime prevention measures. As societies continue to grapple with the persistent challenge of organized crime, this research offers a fresh perspective. It suggests that by viewing criminal networks as complex adaptive systems, we might finally gain the upper hand in this age-old struggle between law and lawlessness.
Author Profile
MSc. Casper van Elteren: A complexity scientist with a craftsman’s soul, Casper van Elteren bridges the gap between theoretical abstraction and tangible reality. By day, he unravels the mysteries of complex systems through mathematical modeling and computational science; by night, he shapes wood and builds split keyboards, proving that understanding comes through creation. With a PhD focus on criminal network disruption and a talent for developing innovative analytical tools, Casper embodies Feynman’s philosophy that true understanding comes from building things from the ground up. Whether he’s developing high-performance computational models or crafting furniture in his workshop, his transdisciplinary approach combines the precision of a scientist with the creativity of an artisan.
Email: Reach out!
Dr. Vítor V. Vasconcelos: Vítor Vasconcelos is an Assistant Professor at the University of Amsterdam, working at the Computational Science Lab at the Informatics Institute. He develops new computational and mathematical models of human collective behavior to anticipate intended and unintended consequences on interventions on complex social systems. Vasconcelos co-leads the POLDER initiative at the Institute for Advanced Study, which aims to help policymakers unravel complex challenges and explore new ways of intervention using simulation, and directs the new MSc Complex Systems and Policy at the Institute for Interdisciplinary Studies. He employs a variety of methods, coupled with experiments and observations, bridging the gap between quantitative and qualitative modelling, and delves into the intricacies of systems involving humans, resources, and governing institutions.
Email: v.v.vasconcelos@uva.nl
Dr. Mike Lees: Michael Lees is an Associate Professor at the University of Amsterdam, where he leads the Computational Science Lab and serves as a principal investigator for complexity at the Institute for Advanced Study. With a PhD from the University of Nottingham and a background in Computer Science/AI from Edinburgh University, his research focuses on using computational techniques to understand complex social systems. Dr. Lees specializes in developing data-driven models and artificial societies to study human behavior and social phenomena. His work spans from data analysis to model development, with applications in various societal challenges including school segregation, urban growth, crowd dynamics, and mental health. Before joining Amsterdam, he was an Assistant Professor at Nanyang Technological University in Singapore.
Email: m.h.lees@uva.nl